Description
Using undesirable output (for example, inaccurate, inappropriate, and user content) for retraining purposes can result in unexpected model behavior.
Why is improper retraining a concern for foundation models?
Repurposing generated output for retraining a model without implementing proper human vetting increases the chances of undesirable outputs to be incorporated into the training or tuning data of the model. In turn, this model can generate even more undesirable output.
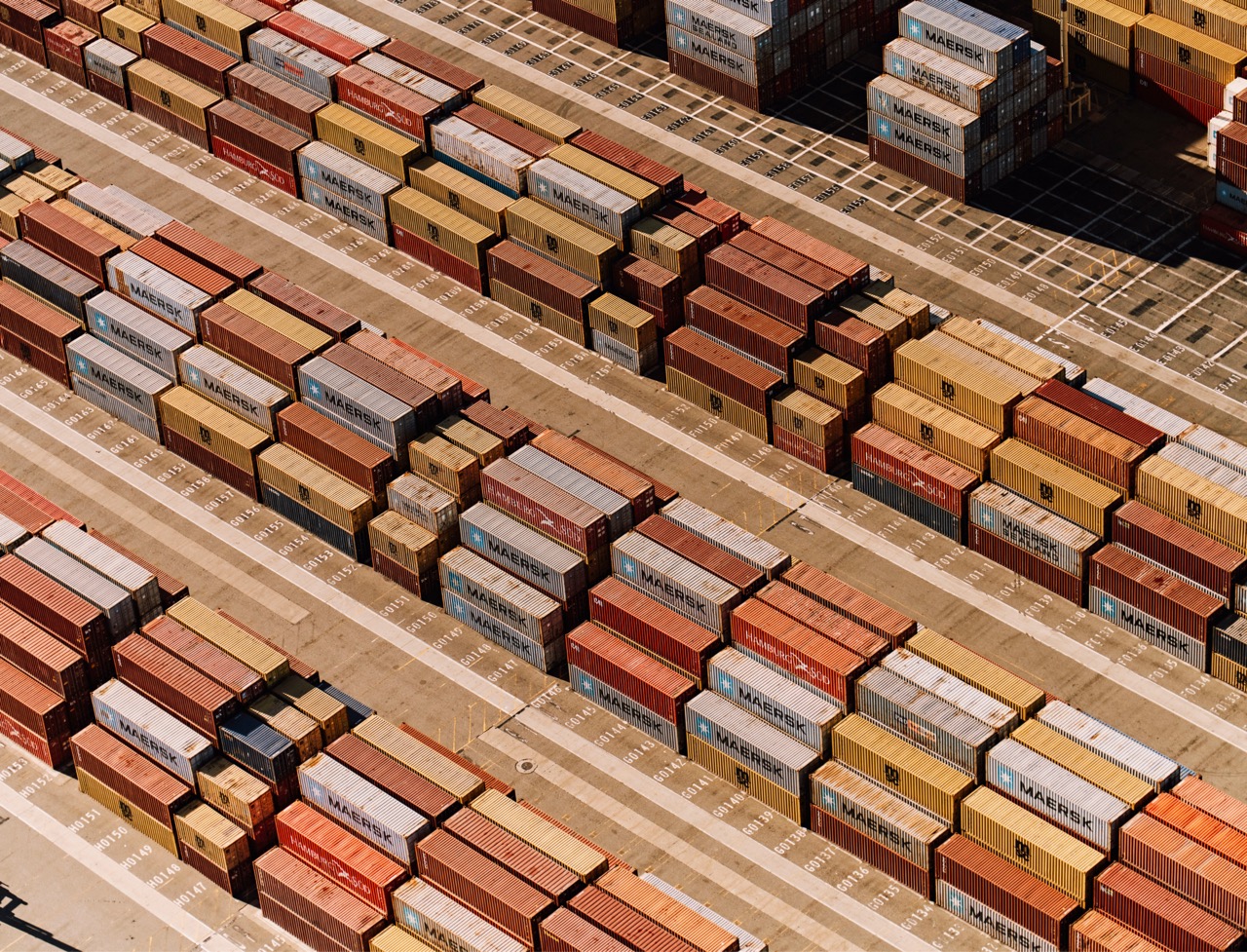
Model collapse due to training using AI-generated content
As stated in the source article, a group of researchers from the UK and Canada investigated the problem of using AI-generated content for training instead of human-generated content. They found that the large language models behind the technology might potentially be trained on other AI-generated content. As generated data continues to spread in droves across the internet it can result ina phenomenon they coined as “model collapse.”
Parent topic: AI risk atlas
We provide examples covered by the press to help explain many of the foundation models' risks. Many of these events covered by the press are either still evolving or have been resolved, and referencing them can help the reader understand the potential risks and work towards mitigations. Highlighting these examples are for illustrative purposes only.